Are diverse datasets medtech’s best path forward?
It seems obvious. If you want medical AI to work on a certain patient population, the model should be trained with those patients in mind.
Yet, in reality, that approach to avoiding bias in algorithm design, model training, and AI evaluation is easier said than done. Frequent MedTech Pulse readers may recall the kidney injury prediction model that worked less well in women—because it was primarily trained on male patient data.
One of the concerns about medical AI which patients, founders, policymakers, and providers return to again and again is this: Bias exists throughout our current healthcare system. How can we design algorithms to make it better? Or, even: How do we keep algorithms from reproducing—or even exacerbating—bias?
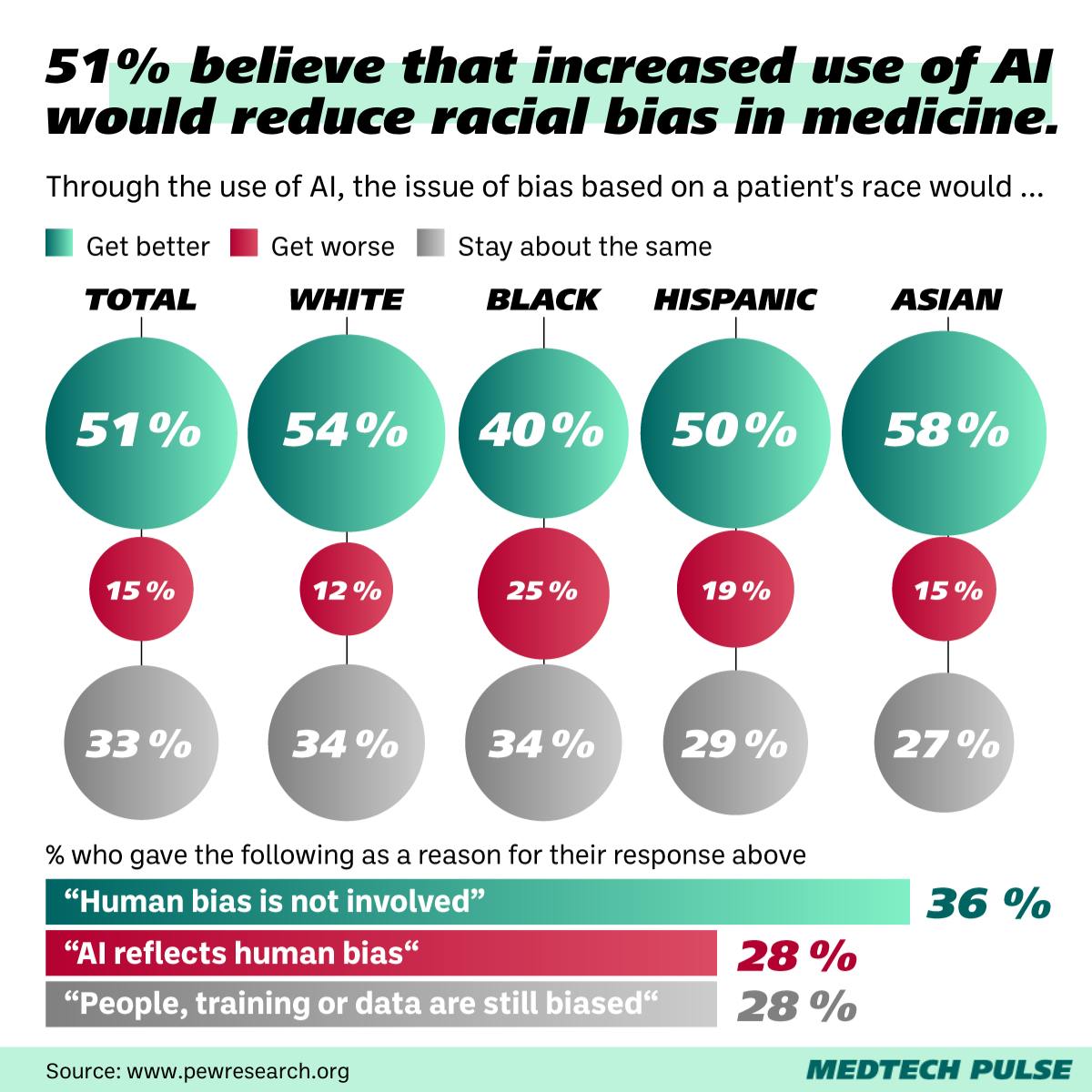
In this edition’s lead article, we’re discussing how AI helps us make unimaginable leaps in precision oncology. With every such tool we develop, the inevitable follow-up questions are: How can we make it work for other conditions? And other patient populations?
Herein lies the central paradox of today’s medical AI paradigm: It currently works best when it is trained on highly-specific datasets, so it isn’t generalizable. However, for it to help the largest amount of patients, we need to build models that are more and more generalizable.
But that doesn’t mean we’re doomed to reproduce medical bias in our industry’s AI solutions. Not if we put our collective minds to it. As I see it, there are three key pathways toward helping medical AI avoid—and even address—existing medical bias.
The first pathway deals with algorithm design. The healthcare industry has long known that medical racism is integrated into many of our existing clinical measures—from pulse oximetry to kidney function calculators. However, physician leaders continue to disagree on whether replacing race as a measure—finding proxies for it—might do more harm than good when we design new algorithms.
The second pathway appears to be making more headway: increasing overall data diversity. After all, without diverse datasets, our AI is limited in the patient populations—and clinical presentations—it can serve. Some of the exciting projects dedicated to improving data diversity for medical AI development include the startup Gradient Health, the landmark expansion of MIT and Philips’ ICU dataset initiative, and the data diversity advocacy coming from the STANDING Together Project.
One clear way we as a medtech community can help push data diversity forward is ensuring our patient-facing digital health tools are accessible and privacy-oriented. To encourage diverse populations to use them—and thus contribute their data. And to that end, we’ll be diving into how privacy plays a role in digital health user uptake in our third Insight this edition.
And increasing the diversity of people represented in datasets goes hand-in-hand with the diversity of people and entities working with—and overseeing—research and AI development. This is the third pathway: improving and increasing independent evaluation. While the new regulation from the EU and US will give us helpful guardrails for managing medical AI, we must also self-govern. That’s why I’m excited by the work of startups like Dandelion Health, which aims to act as an independent evaluator of medical AI.
We’re at an unimaginably fruitful time for medical AI development. Sometimes, It can feel impossible to keep up with all the news of advancements across specialties and sub-industries (though we here at MedTech Pulse strive to help you try). This is why keeping an eye on these questions I’m posing today is so important. We don’t want to hamper our progress—we want to make it sustainable as we keep leaping forward, toward a more efficient and equitable healthcare system for all.