Clinical AI’s complicated relationship with burnout
You’ve heard it. The biggest pitch for clinical use of AI tools? Decreasing clinician workload—and decreasing associated burnout.
The clinical staffing crisis is real. But is AI really going to be the workforce’s saving grace? The answer continues to get muddier.
The story: A new study found that, among radiologists, those using AI tools were more likely to experience burnout.
- Clinician participants were classified based on their workload and their reported acceptance of AI in the workplace.
- Higher prevalence of burnout was found among high and low workload groups as well as high AI acceptance and low AI acceptance groups.
- However, those with low AI acceptance were more likely to experience higher burnout.
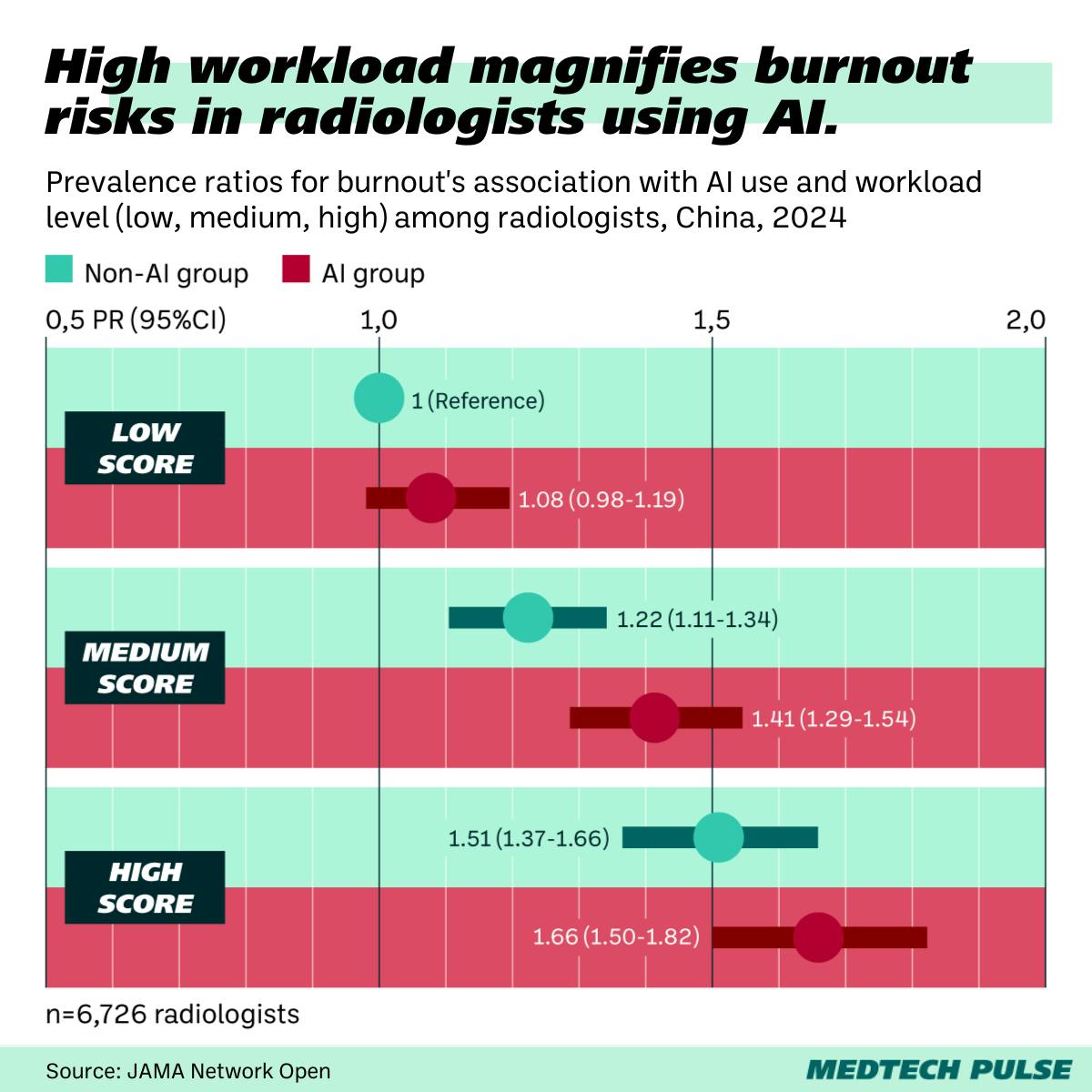
The biggest takeaway feels intuitive: Clinical AI is only helpful when it’s integrated well and lowers the amount of workflow complexity. In some cases, these tools create more workflow complexity, especially for clinicians who don’t understand how to use new tech well.
- Clinical AI not maximizing its usefulness likely also has to do with its design and regulation.
- Most of these tools, especially in radiology, are imaging-related tools evaluated by the FDA under the CADt (computer-aided detection, triage) classification.
- This level is generally considered lower-risk and thus requires fewer hoops to jump through. However, maintaining this classification means that these kinds of tools can’t actually identify what it might have flagged as suspicious on an image, because that would mean it is detecting/diagnosing—which puts it into a higher-risk category for regulatory approval.
- With CADt tools, clinicians still have to read every scan, but the priority order might change based on what the AI found. Radiologists often have to scour an image to try to figure out what the AI might have found to prioritize a certain case, trying to rule out a mistake made by the AI. In short, the tools can actually slow workflows down.
These observations aren’t limited to this study. This mismatch between intention and real-world effects is part of why some hospitals, like Mass General, have actually removed medical imaging AI tools after implementation. In the worst case, clinicians may come to lose trust in their institutions, feeling that their employers have adopted AI tools based on financial incentives, not their practical value.
On the flip side: Studies like this one are an important warning. We can’t go into clinical AI implementation with blind optimism. Yet, there’s still hope that AI can in fact make clinical medicine more human.
- We must learn from the valuable lessons shared by NYU’s largely-successful if limited ongoing ChatGPT pilot program. While OpenAI isn’t a healthcare company, its tools are here to stay in our sector, so we must learn how to make them work for us—not the other way around.
- And we can look forward to more clinician-led ventures for home-grown clinical AI, like the buzzy in-stealth Quadrivia AI.