Could AI improve drug safety surveillance?
Drug and device safety surveillance is a challenging task—especially when, most of the time, only the most extreme adverse events get reported.
What about the adverse events that fly under the radar?
The news: A new research note in JAMA Network Open suggests AI analysis of unstructured data could be the answer.
How the FDA currently tracks adverse events: When a drug hits the market, the FDA keeps watching it through its postmarket surveillance programs. One of the key programs is a data-based tracking system called Sentinel.
- Sentinel’s surveillance analyzes the real-world impacts of FDA-approved drugs. The agency takes this analysis and uses it to adjust drug labels and safety information as well as to convene advisory committees.
- The largest component of the Sentinel System is the Active Risk Identification and Analysis (ARIA) system, which analyzes electronic health record (EHR) data and insurance claims.
However, the ARIA system isn’t always a great fit. It is often deemed insufficient as a way to reliably evaluate safety concerns.
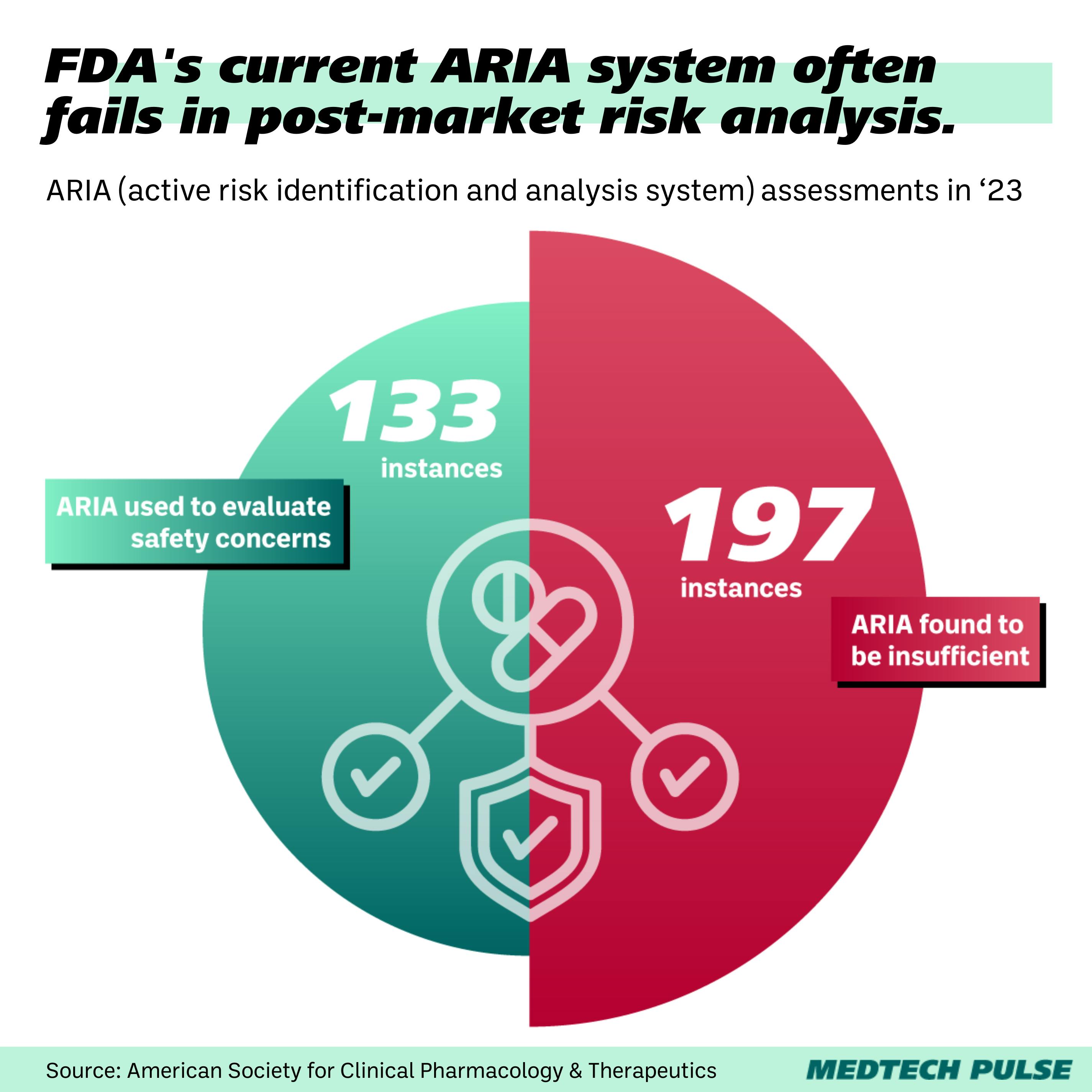
Enter LLMs: With large language models, we can use more unstructured data to identify and analyze adverse events that otherwise wouldn’t end up on the FDA’s desk.
- Think EHR notes and social media posts. The authors of the research note posit that patients may see their doctor or share online that they, for example, had a bloody nose, but such an event wouldn’t be alarming enough to formally report.
- However, while perhaps not being cause for alarm, missing such ‘smaller’ adverse events means we’re likely not getting an accurate picture of the full spectrum of side effects.
So, is this happening?: The FDA has told STAT that they’re already exploring natural language processing and machine learning approaches within their postmarket surveillance systems to integrate more of this unstructured data.
Concerns: However, as with many other LLM applications, there are concerns about bringing these proposals into real-world safety tracking. LLMs are known to hallucinate—or generate false information. In this context, hallucinations could mean over- or under-estimation of safety risks.
Why this matters: While there are many compelling proposals for applying LLMs to medical contexts, this is one of the few that takes on regulatory work.
- As governments around the world take pioneering moves to regulate medical AI, this presents a case for how AI could actually help with medical regulation.
- In the U.S., as Supreme Court decisions threaten to weaken regulatory agencies’ oversight, efficiency and accuracy have never been more important.