Reimagining Imaging: Medical AI takes on radiology
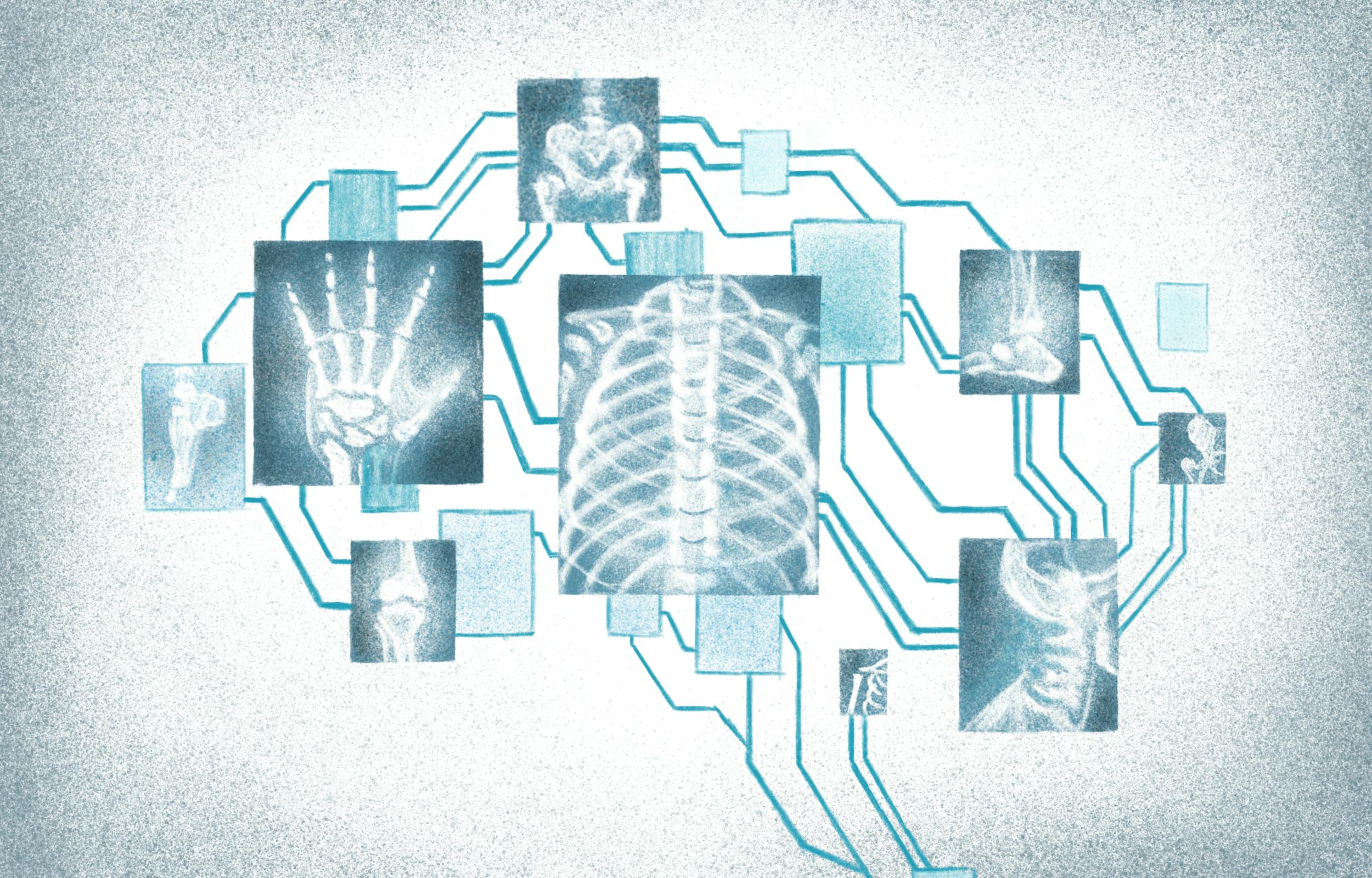
ChatGPT made yet another medical education headline this month when the generative AI tool successfully passed the American radiology board licensing exam.
After an earlier version of OpenAI’s generative AI chatbot—based on GPT-3.5—got close to but ultimately did not pass the board-style radiology exam, the GPT-4-based version exceeded the passing threshold of 70%.
Of course, generative AI tools aren’t going to be replacing real-life physicians anytime (too) soon. In, fact researchers reported being surprised by some of the “very illogical and inaccurate assertions” the AI made on the exam.
But medical AI is making particularly significant impacts in radiology departments, with the trove of complex data in medical imaging being the perfect application for AI-enabled analytics.
The question of data in radiology
Even before today’s generative AI boom, the American College of Radiology’s Data Science Institute found that, in 2020, around 30% of radiologists were using AI in their practice.
It can be easy to understand why. Radiologists manage a wealth of data in many different forms—CT, MRI, X-ray, ultrasound, etcetera.
“There is an avalanche of data, and no one can deal with it,” said radiologist Mathias Goyen, GE HealthCare’s chief medical officer for EMEA.
Artificial intelligence is an excellent solution to that problem—and many radiologists are already catching on. The ACR study found that, of radiologists reporting to use AI in their clinical practice, most were using it to enhance interpretation—especially when it came to detecting critical conditions like intracranial hemorrhage, pulmonary emboli, and mammographic abnormalities. In other words, cases where precision and expediency are of the utmost importance.
But the biggest hurdle to the widespread application of this technology? Data quality of images the algorithms are trained on.
“You put garbage in, you get garbage out,” Goyen said. “There are real challenges in getting accurate and effective data of high-quality that have proper labelling and annotation useful for training.”
As more governments and innovators emphasize interoperability and data-sharing, we hope that improved data standards will serve to train future models on higher-quality datasets.
How will patients and providers benefit from AI-enabled radiology?
For patients, the benefits of AI-enabled radiology are clear. Medical AI makes radiology an incredibly effective tool for advanced screening and risk assessment.
Even the best clinicians run the risk of unintended misdiagnosis when reviewing imagining due to repetitive, high workloads. In fact, the average radiologist reviews 50,000 images in a 12-hour shift. This is up from around 500 images per shift for the average radiologist of 50 years ago.
AI improves interpretation accuracy to lower the risk of misdiagnosis. Plus, AI-enabled imagining interpretation has been shown to also lower clinician reading time by around 40%.
The result? Algorithms help patients get earlier, more accurate diagnoses and personalized interventions than if humans alone are searching for the answers in their scans. This is extremely exciting for the individual—but also for our prospect of building a healthier society, enabled by high-quality preventive care and diagnostics.
AI may be on its way to providing accurate, efficient diagnostic and workflow guidance in medical imaging—sans clinician. That’s why we’re seeing the rise of startups like Eyenuk and Pearl, which both provide autonomous imaging diagnostics. At the moment, these companies primarily aim to help overworked clinicians prioritize which patients need to be seen—an AI-enabled imaging-based triage, so to speak.
Of course, keeping the human intermediary in radiology remains important. It’s different when imaging interpretation comes from an empathetic human face. One that can quell anxieties and answer questions.
Goyen is confident about this, saying: “I don’t want to get my diagnosis from a computer. I want to talk to a real person. If the physician came to the result in this decision based off AI, I love it, it’s great, but I need this human interface. That personal relationship will never go away.”
How will radiology AI impact the healthcare industry as a whole?
The dawn of AI-enabled radiology is yet another factor in the ongoing trend of corporate integration in healthcare.
Especially in the U.S., huge household names like GE are acquiring AI solutions startups to build the technology into their already widespread and trusted radiology offerings. GE’s March 2023 acquisition of Caption Health supports its already $3 billion ultrasound business, bolstered by its 2021 purchase of BK Medical.
As we discussed last year, the European market remains slightly more fragmented, but with the growth of AI startups and the economic downturn, we expect more vertical integration to hit the European market in the future, too—in radiology and beyond.
In the meantime, we’re excited to watch this area of innovation help address radiologists’ burnout, making healthcare a more sustainable industry for them to work in, and improving diagnostics for patients as well.