This wearable sensor can track Parkinson’s progression better than a human can
If you’re an avid MedTech Pulse reader, you’ll know that, in our last edition, we discussed how we’re seeing a turning point for wearables.
This week’s story keeps proving us right.
Clinical diagnosis and monitoring of Parkinson’s Disease just got an upgrade.
A new study from the University of Oxford NeuroMetrology Lab has demonstrated that a wearable sensor paired with a predictive algorithm can accurately track and predict Parkinson’s progression—often better than human observation.
What does this advancement mean for Parkinson’s patients? More effective clinical trials and treatments may be on the way.
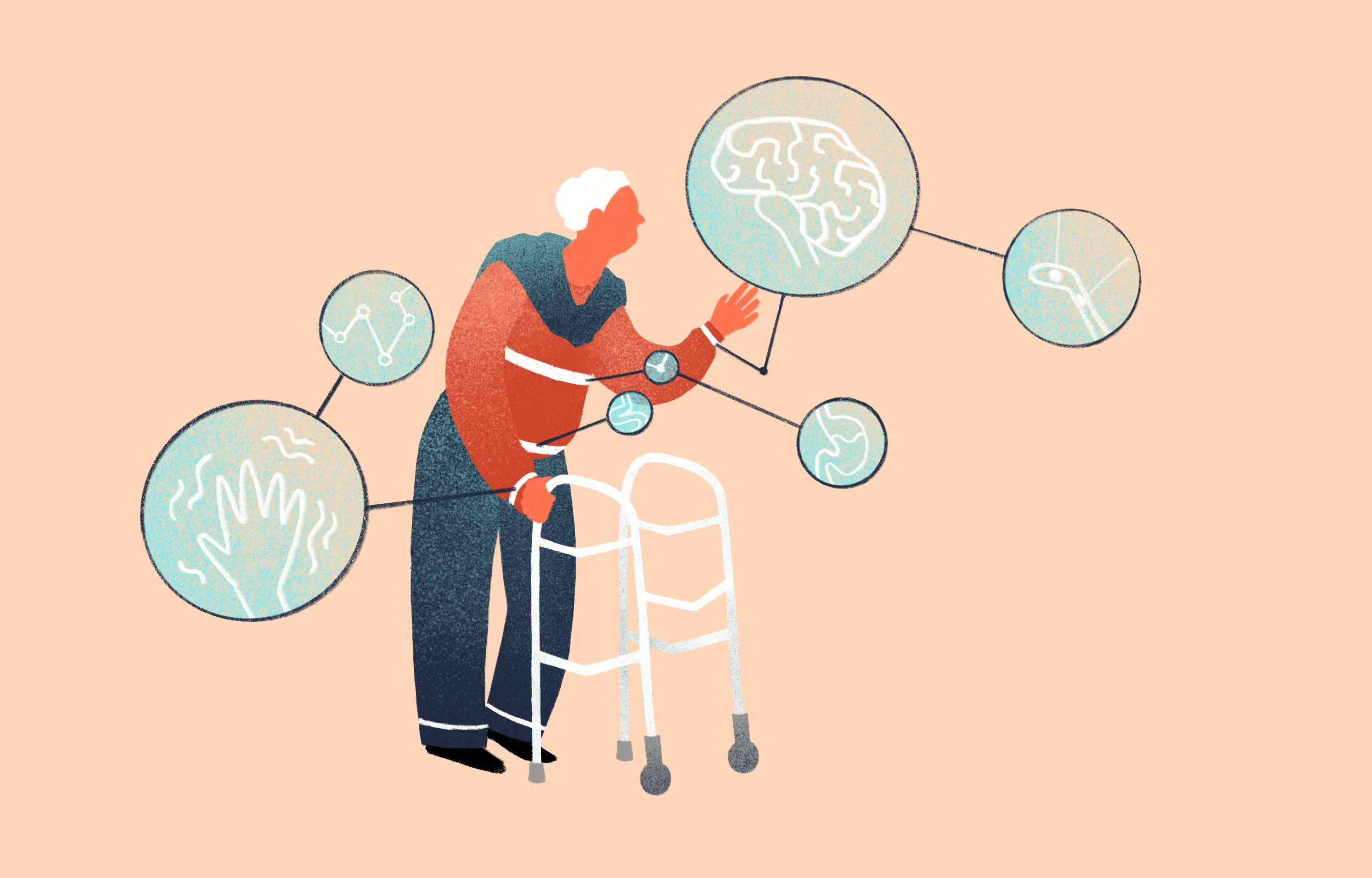
How does the sensor work?
Before this study, previous forays into better Parkinson’s diagnosis have demonstrated that wearable sensors paired with machine learning can lead to more accurate diagnosis. Now, the Oxford lab has taken to honing the method, assessing sensors placed on the torso, wrists, and feet.
The sensors use accelerometer and gyroscope technology—like those found in your smartwatch—to track even a wearer’s most minuscule movements.
They’ve also expanded the method’s applicability. This time, it’s not just about diagnosis—but also tracking disease progression.
The participants wore the sensors while doing walking and standing tasks. They visited the clinic for data collection every three months. Seven different machine learning algorithms—ranging from simple linear regression to Random Forest models—were applied to the data to estimate the clinical rating scale. In the end, the Random Forest model proved to be the most effective.
As a result, the scientists found that their model could track disease progression in just over a year—15 months. In comparison, the traditional human observation-based clinical rating scale would not have captured the change.
The new tracking method can be used alongside existing clinical rating scales to improve the accuracy of diagnosis and tracking of disease progression.
From clinical trial enhancement to potential Parkinson’s treatment
This advancement is exciting for two main reasons: It enables more effective clinical trials and individual patient care.
The current gold standard clinician rating scales are grounded in provider subjectivity. Two providers’ assessments of a patient’s Parkinson’s symptoms may differ in how they score the patient. Plus, the scores are not evenly spaced on a gradient scale—so the difference between a score of 30 and 40 may be different than that between 40 and 50.
This variation can make statistical analysis challenging, causing an unnecessary hurdle in the monitoring of patients enrolled in clinical drug trials for Parkinson’s, for example.
Time is one of the biggest factors in clinical trial success. Earlier and more statistically rigorous detection and tracking of Parkinson’s means clinical trials can evaluate the efficacy of potential treatments more efficiently.
“We have the biomarker,” said lead study author Chrystalina Antoniades. “It’s super exciting. Now we hope to be able to tell you: Is a drug working?”
Plus, when it comes to individual patient treatment, the variability of subjective scoring can lead to treatment delays, which may significantly impact a patient’s shot at keeping symptoms controlled.
Our perspective: Can this analysis turn into a patient-facing tool?
A comparison to Japanese pharmaceutical company Eisai’s new digital dementia business suggests it could.
The company is building out a “dementia ecosystem” based on a patient-user-facing app. Their first product, a risk-scoring tool based on a proprietary prediction algorithm, will help patients self-assess their dementia risk by logging symptoms.
One can imagine a similar tool paired with the Oxford lab’s sensor technology. Of course, correct use of the sensors would likely require a bit more supervision than logging daily mental symptoms in an app. The Oxford team currently insists that their sensors are intended for use with a provider, helping them “get it [the diagnosis] right.”
A similar solution comes from Lotte Healthcare, which is building out an app-based, AI-enabled Alzheimer’s diagnostic tool for seniors. Lotte is working to integrate iMediSync's EEG screening capability into their offering, offering an example of how at-home device-based screening for seniors might look.
These businesses demonstrate trust in seniors and their caretakers to be able to capably take the reins of their healthcare, even if it takes learning a few new digital health tricks.
We’re encouraged by these signals from the market. Empowering older patients with home-based care is a wonderful way to increase access to preventive care and early intervention, especially for those who may find it challenging to come into a clinic frequently.
Plus, with the Oxford team pointing to their technology having applications beyond Parkinson’s—including Alzheimer’s—we’re curious to see what this combination of AI, medtech, and movement science will bring us next.